‘Fragmentomics’ Approach Catches Cancers Earlier In The Blood
By Deborah Borfitz
March 28, 2024 | A machine learning method dubbed A-PLUS (Alu Profile Learning Using Sequencing) recently demonstrated its ability to pick up cancers earlier and with smaller blood draws than is required for whole genome sequencing. This makes possible a future where people might get annual blood draws to check for cancer based on the “fragmentation pattern” of cell-free DNA (cfDNA) circulating in their blood, according to Kamel Lahouel, Ph.D., an assistant professor in the Integrated Cancer Genomics Division of the Translational Genomics Research Institute (TGen), a precision medicine research organization that is part of City of Hope.
The test, developed by researchers at TGen and City of Hope, was “able to identify patients from all the 11 cancers considered, with some types being easier to catch [e.g., esophagus and liver] than others [e.g., breast],” he says. “When looking at all patients with all types together, we were able to catch about half of the cancer patients at a 1% false-positive rate.” Study results published in Science Translational Medicine (DOI: 10.1126/scitranslmed.adi3883).
The main difference between this “fragmentomics” approach and other cfDNA tests is that it amplifies (makes copies) of specific sites of the genome called Alu elements instead of sequencing all spots at a given depth, he explains. Amplifying 350,000 of those sites results in a coverage level on those spots that is about 28 times that achieved by whole genome sequencing.
The rest of the genome is disregarded, adds Lahouel. “The hypothesis here is that there is an enrichment in the difference in fragmentation patterns between cancers and normals at those spots [Alu elements], so it makes sense to zoom on them.”
A-PLUS was trained on how to generate scores using samples from 354 individuals without cancer and 202 patients with cancer, and then had to “learn” the threshold (number) above which it should call cancer and below which it should call normal, Lahouel continues. For this exercise, researchers used a cohort of 958 individuals without cancer and 704 patients with cancer.
Next, they evaluated the performance of the algorithm on an independent cohort, he says. This validation group consisted of 1,793 individuals without cancer and 1,167 patients with cancer.
The input of this machine learning approach consists of a long table of 350,000 entries per patient, says Lahouel. “Roughly speaking, each entry records the number of times an insert in a particular Alu element [locations in DNA] was observed. We start by removing entries [locations] associated with technical noise, ethnicity, gender, etc., to avoid introducing a bias in the classifier.”
In the end, investigators had about 12,000 entries per patient, which they summarized into a table of 60 entries per patient that captured the significant information contained in the initial long table, he says. This set of 60 features was used to generate a “cancer vs. normal” score.
“A score close to 0 indicates normal,” says Lahouel. “A score close to 1 indicates cancer.”
Alu elements are short DNA sequences that are interspersed in the genome and exist only in primates, he notes. “These are repetitive elements in the sense that they are very similar and distributed through the genome; [in fact they] form roughly 10% of the human genome.”
The role of Alu elements in biology and evolution is “an ongoing area of research,” Lahouel says, “but some elements have already been shown to be involved in the regulation of tissue-specific genes.”
For this study, sequencing data was obtained using a PCR-based assay known as Repetitive Element Aneuploidy Sequencing System (RealSeqS). The approach was described in a 2020 article in Proceedings of the National Academy of Sciences (DOI: 10.1073/pnas.1910041117).
Showing ‘Stage Shift’
Major findings from the study were that A-PLUS had an overall sensitivity of 40.5% and specificity of 98.5% and, when combined with aneuploidy in cfDNA and eight common protein biomarkers, those figures rose to 51% and 98.9% respectively, Lahouel reports. “This performance is at least as good as other approaches that have been published.”
Importantly, he adds, the technology used here requires a simpler sequencing workflow and less input DNA than other methods. Most of the cancers tested were also at relatively early stages with very few having distant metastatic lesions.
“Moving forward with such a test will shift the stage of cancer detection in the general population toward early stages, therefore significantly increasing the... success rate of treatment,” says Lahouel.
This summer, the research team will launch a clinical trial in Orange County, California, using another amplicon-based technology developed at TGen and City of Hope that is optimized for the analysis of DNA fragment length, he adds. The prospective study will enroll 15,000 individuals and the objective is to show the “stage shift” in cancer detection by the test when compared to the stages of cancer detection in the general population. The test’s performance will also be evaluated in terms of its estimated sensitivity and specificity.
Liquid biopsy tests can leverage various features and biomarkers of cancer and have the potential to significantly impact treatment outcomes and survival rates, Lahouel says. Choosing or designing the right sequencing approach and technology for analyzing cfDNA “has an impact on the strength of the cancer signal you will measure.”
Leave a comment
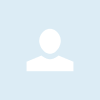
