Bayesian Statistics: An Important Yet Underutilized Paradigm in Rare Disease and Small Population Drug Development
Contributed Commentary by Giles Partington, Phastar
April 26, 2024 | Advances in medical understanding and treatment approaches are driving a revolution in the rare disease space. Approaches such as gene therapies and biologics are offering previously unimagined opportunities to tackle unmet need, changing, and even saving, lives. Yet despite how far we have come in recent years, developing products for rare diseases remains beset with challenges. Chief among these is recruiting enough trial participants to demonstrate efficacy and support approval.
Bayesian statistics, which uses observed events as a way of updating prior beliefs, can be used to generate information equivalent to a number of extra patients being included in a trial. Therefore, some trials have seen robust data brought in through Bayesian statistics equating to an increase of 30% and 2,400% of trial participants. Currently, however, the approach is severely underutilized, in large part due to a lack of understanding of its principles, mechanisms, and advantages.
Giles Partington, Principal Statistician at Phastar explains the basis of Bayesian statistics, looks at its potential applications, particularly in relation to rare disease and small population trials, and outlines the challenges and opportunities on offer.
What Is A Bayesian Statistic?
Bayesian statistics is an approach based on Bayes’ theorem. While frequentist statistics focuses on observing events to attempt to disprove a null hypothesis at a specific p-value and power; Bayesian statistics uses observed events as a way of updating prior beliefs.
A good illustration of how we can use Bayesian statistics is to attempt to calculate the odds of a specific tennis player winning Wimbledon this year. We cannot simply run the same event 100 times and see how many times a player wins to work out their percentage chance this year. We need to consider their previous performances in similar tournaments, how they perform on grass and their recent form. All of this is considered “historic data”.
New information points, like an injury or another player winning all grass-court tournaments in the run-up to Wimbledon, are “observed events” which can be used to change how we look at these factors. Bayesian allows us to incorporate this new information, demonstrating its important adaptive nature. We can now use the historic data as informative priors and update them with the observed events to make our new prediction about the odds of a player winning. If there is no historic information, we can still use vague, non-informative priors as our starting point before updating with observed information (such as assuming all players starting with equal odds of winning).
Clinical trials are likely to have large amount of historic data, including multiple smaller trials and sources of information. These can be used to design informed priors based on a blended method of historic data and expert elicitation – a scientific method to develop well informed, unbiased judgements based on data and past experiences.
The benefits of the adaptive Bayesian approach have been recognised by regulators. The FDA says Bayesian analysis “brings to bear the extra, relevant, prior information” which can aid decision making. The EMA has also highlighted the potential uses of Bayesian approaches, particularly in early clinical development and when there are rare indications.
Applications For Drug Development
Bayesian methods allow us to utilise information from smaller trials or other sources, including healthcare professional (HCP) opinion. These sources can then be combined to reduce reliance on larger sample sizes.
This is particularly important in rare disease or small population trials where the number of eligible candidates complicates recruitment, resulting in weaker powered trials and less reliable outcomes.
For example, Juvenile Localised Scleroderma (JLS) has an incidence of about 3.4 per 10,000 children. The first line treatment has poor tolerability and there is limited evidence around efficacy for the second-line treatment. A frequentist non-inferiority trial would require 320 patients. With around 43 new patients per year in the UK, this would take about 15 years to recruit. However, if we start to capture current understanding of the treatments, we can form the basis for a Bayesian trial.
With a Bayesian method, we can set up priors that can be used to simulate trial outcomes multiple times. These simulations can be used to run hundreds of iterations before any patients are even recruited. By averaging the results of these simulations, we can calculate a sample size that allows for reduced patient numbers and achieve more reliable outcomes which are not restricted by power calculations.
A Bayesian framework is also useful for adaptive designs because it can accommodate changes to dosing during recruitment. For example, a trial with five potential drug dosages can be analysed after each dosage has 20 patients recruited to it. The worst two performing doses can then be dropped from further recruitment. While a frequentist framework would need to increase sample sizes to avoid false positives, a Bayesian framework allows multiple testing without issue.
The Current State Of Play
Despite its advantages and regulatory backing, Bayesian statistics remains underutilised. A 2022 review of methods to overcome restricted populations within rare disease and small population trials identified 64 eligible studies which had taken place since 2010. Of those, only four used Bayesian methods. The remaining 60 were frequentist.
The review, which was published in the Journal of Clinical Epidemiology, found frequentist trials had a planned power of 72-90% but reported recruiting a mean of 6.6% fewer patients than planned. Most used standard Type I errors – 52 used an error rate of 5% and one used a 1% error rate. The average standardized effect was high at 0.7%, but 50% of trials missed this assumed level.
Of the Bayesian trials, three used informed priors – two in design and one in analysis. Methods used to inform priors included historical data and expert consensus. The Bayesian trials would have required between 30% and 2,400% more participants to power the study in a frequentist framework.
The review concluded that Bayesian trials required lower sample size through use of informative priors. Most frequentists did not achieve their target sample size.1 While Bayesian methods offer promising solutions for such trials, they are underutilized.
The Challenges And Opportunities Of A Bayesian Approach
Bayesian statistics does not change the basic rules of a clinical trial, but the approach does need to be integrated from the start. The prior distributions, data to be collected, and type of analysis need to be selected and validated in advance.
Some elements of a Bayesian approach can also be more complex than frequentist trials, for example, expert opinion consensus collection. If possible, it is best to elicit a single prior that represents the collective belief of experts based on discussion of their individual priors. This requires careful and robust facilitation, an adaptive process and for all experts to be provided with relevant information. All of this can mean longer set up times.
However, the opportunities for overall efficiency and effectiveness offered by Bayesian statistics far outweigh the disadvantages of initial set up time.
Ultimately, understanding treatment efficacy and safety is the goal of a clinical trial but it is not always possible to run a well powered, randomised controlled trial to detect a treatment difference in rare diseases. Bayesian statistics allows us to use existing evidence and expert opinion to quantify uncertainty and inform trial design.
Giles Partington is a Principal Statistician at Phastar consulting multiple clients on trial design, especially in early phase studies. He also works as part of the Bayesian elicitation research team within the company. Previously he did a pre-doctoral fellowship with Imperial Clinical Trials Unit focusing on the usage of Bayesian statistics for rare disease/small population trials. He can be contacted at giles.partington@phastar.com.
Leave a comment
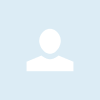
