Revolutionizing Clinical Trials with Generative AI
Contributed Commentary by Wing Lon Ng, Director of AI Engineering, IQVIA
July 19, 2024 | The rapid advancements in artificial intelligence, particularly generative AI (GenAI), are enabling broader technology acceleration and driving a significant transformation in the healthcare industry. This democratization of AI capabilities opens the door to widespread innovation across clinical trials and drug development and represents a unique change in thinking compared to previous technological advancements.
AI streamlines and automates numerous processes in clinical research and trials. It enhances patient recruitment, improves data analysis and enables predictive modeling, all of which are key areas of impact. AI can analyze vast amounts of data much faster than humans to identify potential patients. Algorithms can analyze complex datasets, identify patterns, and predict outcomes much faster than humans.
The addition of GenAI to this technology mix is proving particularly valuable in accelerating trial design and execution. For example, GenAI can rapidly generate draft protocols by analyzing historical trial data, automating document creation and enabling real-time trial adjustments based on emerging data. These AI-powered capabilities are cutting down planning time from days to minutes for many trial-related tasks.
Beyond these efficiency gains, GenAI facilitates smarter, data-driven decisions throughout the clinical trial lifecycle. AI can simulate different trial scenarios, recommend optimal strategies and surface key insights from massive datasets. This allows sponsors to make faster, more informed choices about trial design, site selection, and patient recruitment.
Most importantly, GenAI has the potential to make clinical trials more responsive to patient needs. AI-powered matching can connect patients to highly relevant trials. Personalized treatment plans can be developed based on individual patient data. Additionally, AI-enabled remote monitoring allows for less disruptive trial participation.
Addressing Challenges
While the potential benefits of implementing GenAI in clinical trials are significant, several crucial hurdles must be overcome to ensure the safe and effective use of this technology in such a critical domain.
Data quality and standardization represent a primary concern. Clinical trial data often comes from diverse sources, with varying formats and levels of completeness. For GenAI to function effectively, it requires high-quality, consistently formatted data. Achieving this standardization across different healthcare systems, research institutions and geographical regions is a formidable task that requires coordination and investment.
Regulatory compliance adds another layer of complexity. The pharmaceutical and healthcare industries are heavily regulated, and for good reason—patient safety is paramount. Integrating GenAI into clinical trial processes must align with existing regulatory frameworks, which may not yet be fully adapted to these emerging technologies. This necessitates ongoing dialogue between AI developers, healthcare professionals and regulatory bodies to establish proper guidelines and standards.
The risk of AI "hallucinations" is particularly concerning in clinical trials. These instances, where AI generates plausible but incorrect information, could lead to serious consequences if not properly identified and managed. In a field where decisions can directly impact patient health and the validity of scientific research, even small errors can have significant repercussions.
To address these challenges, implementing robust safeguards is crucial and requires a multidisciplinary approach, involving collaboration between AI developers, healthcare professionals, ethicists and regulatory experts. Cross-checking mechanisms, where AI-generated information is verified against multiple reliable sources, can help catch potential errors or inconsistencies.
The Importance of AI “Explainability”
Mandatory explanations for AI recommendations provide transparency into the AI's decision-making process and help human experts evaluate the suggestions' validity. As organizations increasingly rely on AI for critical decisions, there is a growing need for these systems to be not only accurate, but also explainable.
Explainable AI (xAI) refers to the ability of these technologies to clearly communicate to users why a particular decision or outcome was reached. Explainability has become an important factor. The opacity of certain machine learning models, dubbed “black boxes,” is important, especially in understanding how AI models arrive at decisions. Black box models are historically closed, powered by deep neural networks and sophisticated algorithms. By nature, the outputs are accurate but not explained. While this is acceptable for many applications, when it comes to predictive modelling and diagnostics in clinical trials, drug development and healthcare, it is imperative that the decisions made by AI are understood, transparent, and can be verified. Understanding the reasoning behind a certain diagnosis or prognosis is not only a matter of curiosity in important medical settings when judgments might be life or death. It is a vital necessity for trust and responsibility.
It’s here that "white box" models that not only deliver correct predictions but also provide insights into how these predictions are created are key. This multidisciplinary strategy combining advances in model interpretability, feature importance analysis, and a commitment to ethical AI practices is required to achieve this transparency.
The “why” is crucial to achieve both explainability and accuracy in AI to build user trust and identify potential biases effectively. Explainability and interpretability provide clearer insights into how AI models arrive at their conclusions, making it easier for human experts to validate and trust the output.
By carefully navigating these hurdles and implementing appropriate safeguards, the integration of GenAI into clinical trials can be achieved responsibly, potentially leading to more efficient, accurate, and innovative research processes in healthcare.
Human-in-the-Loop Remains Key
While GenAI can process vast amounts of data and identify patterns beyond human capacity, the final decisions in clinical trials should always involve human judgment. This "human-in-the-loop" approach is essential as it ensures that ethical considerations, contextual nuances, and the potential real-world implications of decisions are thoroughly evaluated.
It must be emphasized that the goal is to augment rather than replace human expertise: AI recommendations still require human review and approval, which is important when combining the strengths of machine learning with human judgment. It recognizes that while AI systems can process vast amounts of data and identify patterns with remarkable speed and accuracy, they lack the nuanced understanding, ethical reasoning, and contextual awareness that humans possess.
Integrating AI recommendations with human review and approval leverages the strengths of both machine learning and human judgment. AI can rapidly analyze complex datasets, generate insights and propose solutions, while humans can evaluate these recommendations, consider broader implications and make informed decisions. Importantly, this collaborative approach also facilitates continuous improvement of AI systems. Human feedback and corrections refine and enhance AI models over time, benefiting both the technology and its users.
The Future of Clinical Research
The impact of AI extends beyond clinical trials to the broader field of medical research. Improved diagnostics, personalized treatments and accelerated drug discovery are key areas where AI is driving advancements. Virtual modeling of drug interactions, for instance, can significantly speed up the initial stages of pharmaceutical research.
As GenAI capabilities continue to advance, we can expect to see even greater impacts on drug discovery, diagnostics and personalized medicine. Despite the remaining challenges, integrating GenAI into clinical trials promises to accelerate medical innovation and enhance patient outcomes.
The integration of GenAI into clinical trials promises to benefit all stakeholders. For sponsors, it offers real-time updates and detailed reporting. For site staff, it reduces repetitive workloads. Regulatory authorities benefit from enhanced trial quality and reliability. Most importantly, AI can help identify the most suitable patients for trials, develop personalized treatment plans and enable more accessible clinical trials and effective remote patient monitoring.
Wing Lon Ng has over 20 years of experience in data science and machine learning. As a trained statistician and econometrician, with a PhD in Economics, he has a strong background in applied data science in academia and industry, with over 50 research articles published in international journals and conferences. Wing Lon currently leads IQVIA’s ACOE MLE team as the Director of AI Engineering, specializing in clinical trial strategy planning and automation. He can be reached at winglon.ng@iqvia.com.
Leave a comment
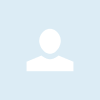
