Youth Diabetes Researchers Invited to Dip Into POND, Make a Hypothesis
By Deborah Borfitz
July 30, 2024 | Soaring rates of youth prediabetes and diabetes over the past few decades have prompted a multidisciplinary team of experts from the Icahn School of Medicine at Mount Sinai (ISMMS) to create a dataset unifying potentially important clues about how to personalize interventions and devise better prevention strategies. Type 2 diabetes and its precursor, prediabetes, are complicated, seemingly intractable health conditions, in part because interventions haven’t been tailored to the needs of different subgroups of patients, according to Nita Vangeepuram, M.D., MPH, associate professor of pediatrics, population health science and policy, and environmental medicine and climate science.
It wasn’t until early in the 21st century that type 2 diabetes was even thought of as a condition afflicting youth, she says. That realization has been attributed to growing rates of obesity and declines in healthy eating and activity levels in children and adolescents.
Given that the combinations of disease risk factors can differ significantly from person to person, it follows that more nuanced treatment regimens are needed to stem the epidemic, Vangeepuram says. However, until now, progress has been hampered by the lack of a comprehensive and accessible dataset providing a detailed view of the vulnerabilities and trends.
That all changed with the recent launch of the study’s dataset and publicly available POND (prediabetes/diabetes in youth online dashboard), both enabled by the availability of National Health and Nutrition Examination Survey (NHANES) data collected from 1999 to 2018 by the Centers for Disease Control and Prevention. It was a multi-year, multidisciplinary effort combining the expertise of Vangeepuram, a clinician and child health equity researcher, with the machine learning knowhow of Gaurav Pandey, Ph.D. and epidemiology knowledge of Bian Liu, Ph.D.
More than two dozen variables—race and ethnicity, health insurance, body mass index (BMI), and screen time among them—were identified as being significantly correlated with youth prediabetes and diabetes in an article about the dataset and POND that was published recently in JMIR Public Health and Surveillance ( DOI: 10.2196/53330). Across different statistical and machine learning methods, “the same story played out over and over again,” notes Vangeepuram.
Known and Novel Associations
The analysis found both known and novel variables that matter in terms of disease risk. Most surprising, though, is “that there were associations across all the different domains [sociodemographic, health status, diet, and lifestyle behaviors] included in the dataset,” Vangeepuram says. “This makes the case that we need to do more research to figure out what groups are at risk, which risk factors are important for whom, and what we do about it.”
A pair of case studies included in the published paper point to the potential of the dataset for translational studies, says Liu, associate professor of population health science and policy, and environmental medicine and climate science at ISMMS. One identified 27 individual variables associated with prediabetes and diabetes status and the other predicted that status using machine learning.
Both the statistical bivariate analyses and a machine learning framework known as Ensemble Integration (El) identified 27 risk-associated variables, 16 of which overlapped: gender, food stamps, race and ethnicity, and insurance (sociodemographic); weight, height, waist circumference, BMI, taking prescription drugs, hypertension, and general health (health status); total protein, oils, fruits, and solid fat consumed over 24 hours (diet); and screen time (other lifestyle behaviors). The El approach also identified 11 additional predictive variables, including some known (e.g., meat and fruit intake and family income) and other less recognized factors (e.g., number of rooms in homes), the study team reports.
It is believed to be the first such dataset of its kind for youth prediabetes and diabetes, says Liu. A preliminary search turned up only collections focused on adult-onset diabetes or comprised essentially of genomic data.
Inspiration for creating POND comes from web portals in the genomics field, such as The Cancer Genome Atlas and Genomics 2 Proteins Portal, says Pandey, Ph.D., associate professor of genetics and genomic sciences, and artificial intelligence and human health at ISMMS. However, unlike POND, these online platforms aren’t tied to the daily life realities and risk factors of diabetes among youth.
NHANES data, while available on the internet, requires some expert knowledge and extensive effort to properly analyze and be of practical value to most would-be users, Liu says. The Mount Sinai team spent several years cleaning and harmonizing the data, selecting the variables for the case studies, and getting the information into a user-friendly format for the POND web portal so it would be of value to other hypothesis-testing researchers as well as healthcare professionals and the public.
Through this part of the work, the team aims to illustrate the utility of data sharing, transparent methodology and code, and public data portals to the epidemiology and public health communities, says Pandey.
Personalizing Prevention Interventions
It is well known that type 2 diabetes and prediabetes disproportionately affect racially and ethnically minoritized populations across the lifespan, says Vangeepuram. These are also understood to be multifactorial conditions, although not all the risk-imparting variables have been identified, nor the extent of their impact on different subpopulations.
Although type 2 diabetes has conventionally been thought of as an adult disease, rising prevalence of the disease and related metabolic conditions in youth has drawn attention to the differing burden of complications they face, says Liu. Preventing the progression of prediabetes into full-blown diabetes with all its unwelcome health consequences was one of the initial motivations for creating the dataset and POND.
Vangeepuram sees all this firsthand in her clinical practice. “When you diagnose these conditions early on in life, there is a much greater chance that [patients will] develop diabetes-related complications, of which there are many—eye disease, kidney disease, amputations, and cardiovascular risks—so the earlier you can intervene the better.”
Interventions to date have been “more cookie cutter” than personalized to patients based on their differing characteristics, Vangeepuram continues, adding that she is particularly excited to explore subgroup differences to inform prevention efforts. These are not the kinds of things that are easily addressed in the context of a 15-minute well child visit. With so many topics to cover, ranging from needed vaccines and issues around regular growth and development, often little time remains for pediatricians to delve into a child’s struggle with weight and risk of diabetes, and other metabolic conditions.
Translation Potential
The work of the Mount Sinai team began with the processing of NHANES data from 10 survey cycles (1999-2018), which yielded a dataset covering 15,149 youths with known prediabetes or diabetes status (yes or no) based on fasting plasma glucose and hemoglobin A1C biomarkers, says Liu. They then selected 27 potentially relevant NHANES questionnaires grouped by domain—sociodemographic (demographic, socioeconomic, and social determinants of health variables such as age, gender, poverty status, and food security), health status (e.g., blood pressure, total cholesterol, and BMI), diet (e.g., meals eaten per week and added sugar intake), and other lifestyle behaviors (physical activity, screen time, and exposure to secondhand smoke).
From the selected questionnaires, investigators next extracted 95 potentially relevant variables, and through statistical analyses and machine learning determined which were most associated with youth prediabetes and diabetes risk. Nearly a year was spent creating POND to enable any user with an internet connection to navigate, visualize, and download the data themselves, says Pandey, adding that the user-friendly web portal includes the code used to generate the data, in addition to the two case studies. The code to build POND is also publicly available on the portal, which the team hopes will enable similar systems in public health, epidemiology, and beyond.
Since the study population was derived from NHANES, which is “quite representative of the problem and the population” across the country, Pandey says, the utility of the dataset and POND should remain for years to come. “Our goal is to enable data-driven discovery in the field like never before.”
POND isn’t expected to require much IT support from his lab, says Pandey. The team is also happy to field questions via email, as needed, to facilitate usage by outside groups looking to help fill the research gaps.
The dataset is not without its limitations, Liu says, since it was drawn from a cross-sectional survey. The prediabetes and diabetes status and related variables included in the dataset provide only snapshots of youth in the U.S. over the available, two-year NHANES survey cycles, making the identified associations best suited for hypothesis generation purposes that get tested in prospective longitudinal and randomized trials.
Vangeepuram’s focus is squarely on the important, persistent, and worsening public health problem these childhood conditions represent across entire lifespans. Youth prediabetes and diabetes have huge implications for the health and economy of the nation, she says, so “we have to use the information we have to start figuring out what we can do about it.”
Given the transparency around the process used to develop the dataset and web portal these resources could be useful for different datasets for other health topics, she adds. For example, the methodology might be used to construct a similar dataset and portal specific to youth mental health to better prevent and treat depression, anxiety, and suicidal ideation.
Leave a comment
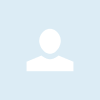
