Follow Five Best-in-Class Technology Practices for Improved Data Management Outcomes
Contributed Commentary by Jennifer Duff, Zelta Clinical Trials Solutions
August 13, 2024 | In today's data-driven world, clinical trials rely heavily on effective data management to ensure the accuracy and integrity of research findings. Improved data management outcomes ultimately benefit the primary beneficiary—the patient.
Best-in-class refers to the superior product within a specific category of hardware or software. Best-in-class is often confused with the best product on the market. The difference between the two is that best-in-class describes the best product within a specific category or price range, while the best product on the market covers all classes and price ranges. Source: Capterra
Clinical trial technology covers all solutions, from patient recruitment and monitoring to data management and data acquisition, including trial, safety, and regulatory operations. This inclusive, end-to-end ecosystem of possible solutions also includes machine learning, artificial intelligence, advanced automation, and visualization converging technologies.
In my experience, these five best-in-class technology approaches improve data management outcomes in clinical trials executed by contract research organizations, sponsors, academics, and registries.
A Thinking Ecosystem
Clinical research is constantly evolving, and while it’s evolving, so is technology. In the clinical data management space, applying a structured approach offers two options:
- Single vendor: A company may invest significantly when implementing a single vendor’s technology and then, over time, customize that ecosystem. The customized system becomes so entrenched in the company’s processes that it’s difficult for them to change. They may face challenges of pricing, special features, and eventually, vendor management or integration, leading to increased labor expenses.
- Ecosystem: Most people encounter the ecosystem mindset daily with their smartphone applications, selected for personal interests and convenience—for example, banking, music, airlines, games, and healthcare. Each app performs a specific function and exists within the smartphone’s ecosystem. Best-in-class technology for clinical trials operates similarly to the smartphone’s ecosystem. Multiple software providers specialize in or focus on specific areas and leverage their experience to develop more efficient market solutions tailored to user needs.
With so many vendors involved, the trial may require collaboration among these players, such as for service questions or to optimize login and access seamlessly with workflow. Quality best-in-class vendors generally are open to working together to meet the needs of the clinical trial with an eye to minimal user burden in accessing and leveraging the various solutions within their workflow.
CROs and sponsors can improve decision-making with either a single-vendor or an ecosystem approach. Either way, they must explore four areas with their technology vendors to be successful:
- Usability — Is the technology user-friendly? Are references available? How does the vendor support the product? Does the system support real-time reporting of relevant measures?
- Technology and Innovation — Are there timely updates to the platform? Are there open and accessible APIs? At what frequency can data flow—at least a minimum of near real-time?
- Functionality — Does it meet your needs, as evidenced by scaled implementation, and not just promise? Can you see it in production in a demo? Can you access a sandbox to explore capability?
- Quality and Compliance — Does the technology have these components: Full QMS, ISO-Certified, HIPAA-Enabled, follows GDPR guidance, 21 CF Part 11 compliant? Will the vendor allow audits?
Applying the right approach and fully exploring the usability, technology, functionality, and compliance aspects of both vendor and technology are vital to success.
The Efficiency Of eConsent
The efficacy and value of eConsent depend on understanding whether the technology fits the clinical trial’s scope, site experiences, and choices that make sense for the stakeholders that matter.
From the sponsor’s perspective, eConsent offers a centralization advantage for building and deploying consent packets to sites. This approach provides more control over consent and real-time access to consent progress, issues, and opportunities.
However, the drawback is that eConsent adds another technology for the sites to learn and maintain. Some local sites won’t approve any consent approach over something already in place at their respective locations. Those sites implementing the eConsent technology must also license and maintain it; thus, sites can be primary decision-makers on the adoption of eConsent.
Yet, with a primary focus on the study participants, eConsent enables them to access that consent remotely, whenever and wherever they are. In some cases, the technology enables them to pose questions and interact with someone for support when making those consent decisions.
Technology is an opportunity to involve the sites and study participants earlier in decisions that offer flexibility and choice. Offering site choices enables them to pivot from eConsent or wet ink solutions. For example, sites may use their technology but ultimately supply the consent through a sponsor-offered portal. eConsent is one opportunity to improve collaboration between clinical operations and data management in the clinical trial setting.
Beyond APIs
The combination of open APIs and industry standards can achieve interoperability and demonstrate the value of integration over data entry.
Application programming interfaces (APIs) enable applications and systems to communicate by exchanging data and enabling functionalities. Within the clinical data management space, open APIs pose one of the most exciting opportunities for clinical trials.
Today's data standards and transfer formats support interoperability across the clinical and health space. The list below identifies the standards most often used for data exchange in a clinical trial setting.
- CDASH: Clinical Data Acquisition Standards Harmonization
CDASH is a standard way of collecting clinical data consistently across studies and sponsors so that those involved in the research know where the information is and how it is stored.
- ODM: Original Data Manufacturer
ODM, an operational data model used to define and standardize metadata, is a blueprint of where information lives behind the scenes.
- USDM: Unified Study Definitions Model
USDM helps with alignment and, in conjunction with CDASH and ODM, enables a standard across solutions that touch different areas of clinical trials.
- XML: Extensible Markup Language
XML stores data in a format that can be stored, searched, and shared. Most clinical trial solutions can send or condense data in XML.
- JSON: JavaScript Object Notation
JSON is the standard approach for storing and transporting data.
- FHIR: HL7 FHIR (Fast Healthcare Interoperability Resources)
This standard defines the exchange of healthcare information between different computer systems, no matter how it is stored. It can tap into the electronic health record because of its standard output.
With an ecosystem approach, even without the daily use of multiple platforms, data comes in from somewhere along the end-to-end chain of the clinical trial. Automated transfer via APIs may or may not be the appropriate way to integrate clinical trial technology.
When deciding if APIs are the best approach, organizations must consider how vendors maintain APIs in their technology. They should also define how often they transfer data and the volume and type of data exchanged based on business needs.
Empowering Change
As with most things in business and life, ultimately clinical trials are about people and data. The human element is critical to managing and driving success, timelines, and quality. Well-designed processes should ensure the desired outcomes and use technology to improve outcomes.
While the three parts of change management (people, process, and technology) must come together, the people piece is most critical to managing success. Current research (Phillips, J., and Klein, J.D., 2023) identifies these three steps for effective change management.
- Begin change management early, even before the team selects and deploys the technology, and include the site/user in this process.
- Involve the user to ensure the change is something the user wants instead of a change handed down by executive management.
- Change the thought process on change management as something that starts and stops at a certain point. It takes time and continuous reinforcement around education, reward, highlighting impact, and capturing user experience stories.
Change management doesn’t always need external help. Internal stakeholders can and should often drive vital components of change because change typically links to the users. The internal leaders also gain experience and ultimately control the outcomes.
Yet, consultants can offer an outside perspective on the situation, generate new ways to communicate at all levels, introduce information from the field, and bring focus and urgency to change management as a continuous process. Leverage outside expertise to supplement internal capabilities.
Artificial Intelligence (AI) And Machine Learning In Clinical Research
AI and machine learning solutions applied to data management challenges in clinical trial research require a structured approach. The two most familiar models are the Large Language Model (LLM) and the Small Language Model (SLM).
Each model offers specific benefits and challenges with clinical trial data management. LLMs are less controlled and require more training resources, but they excel at analysis and creating content (think Chat GPT). SLMs are more closely supervised as they train and require less data. They can solve precise problems, such as medical coding or lab conversion.
- To address the challenges of using AI or machine learning in data management for clinical trials, organizations should:
- Identify the problems, understand the expected results, and measure return on investment.
- Work AI and machine learning into current workflows for efficiency in decision-making.
- Follow the most recent guidance from the United States and the European Union.
- Avoid bias in the data, no matter the data source.
- Ask questions about AI training.
- Make sure data use rights are understood and part of the commercial agreement.
- Include a feedback loop in the model and be prepared to invest in the evolution of AI/ML.
Improvement in data management outcomes with best-in-class technologies is not only a technology issue. Leaders must think about technology holistically. While standards are necessary and technology approaches are essential, it’s vital that CROs, sponsors, and all organizations involved in clinical trials factor in people for the process change and create the right environment for them to succeed.
Jennifer Duff is Executive Vice President and General Manager of Zelta Clinical Trials Solutions at Merative. She has more than 26 years of experience in the Life Sciences industry with a specialization in enabling and scaling industry-leading services and technology solutions for biopharma clients. Jennifer has collaborated with teams across the Life Sciences industry, from large to mid-sized companies, as well as many of the major technology providers over her career. She has an MBA in Biotechnology and Healthcare Management. She can be reached at Jennifer.Duff@merative.com.
Leave a comment
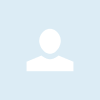
