AI Beyond the Hype: A Game-Changer for Scaling Clinical Trials Without Sacrificing Quality
Contributed Commentary by Henry Lin, Emmes Group
December 13, 2024 | In the past year, the rise of generative AI has created much buzz within the life sciences industry. This technology promises to shorten the lengthy timelines typically seen when bringing new therapies to market, providing a glimmer of hope (perhaps hype?) for an industry known for its methodical pace. The challenge is to harness AI’s capabilities safely, securely, and effectively to accelerate every phase of the drug discovery and development lifecycle. And while much of the conversation has centered on AI’s potential, its tangible benefits in clinical trial design and execution are already reshaping how trials are conducted, improving efficiencies and timelines.
Tech-enabled CROs are leading this transformation by integrating AI into traditionally manual processes—protocol development, site selection, study setup, data cleaning, and report generation. These applications of AI go beyond speeding up timelines; they are enhancing quality and consistency in ways that manual processes alone could not.
Beyond operational gains, there is growing interest in using AI-driven insights from clinical trial data to make better decisions for future studies. AI empowers clinical teams with unprecedented access to real-time data, allowing for on-the-fly adjustments and informed decision-making. This capability spans from protocol optimization and trial monitoring to analyzing patient-reported data and wearables, allowing AI-driven insights to drive the future of clinical trials. Ultimately, this evolution is more than a logistical benefit—it represents real-world value for sponsors and, most importantly, for patients who stand to benefit from faster and more effective studies.
One area where AI shows remarkable promise is in deploying large language models (LLMs) to automate document-intensive tasks, like data extraction and document generation. LLMs can easily create and digitize complex documents, including study protocols, reports, and informed consent forms. By streamlining documentation, these models are reducing development timelines and simplifying complex administrative tasks that have historically been slow and labor-intensive.
Consider the example of protocol optimization: analyzing historical data enables researchers to refine critical inputs such as inclusion/exclusion criteria, visit frequency, and monitoring protocols. Through predictive algorithms, AI can optimize electronic case report forms (eCRFs) and validation checks for variables in record time. This shift shortens the process from months to weeks and allows studies to meet standards like Clinical Data Acquisition Standards Harmonization (CDASH) upfront, ensuring consistent data formats across studies. This proactive standardization significantly enhances efficiency, as data exports are immediately ready for analysis, eliminating the need for later re-formatting.
AI’s strength lies in processing vast amounts of data at speeds unmatched by human teams, allowing clinical teams to focus on more nuanced aspects of trial management. Rather than spending countless hours on spreadsheets and hunting down inconsistencies, Clinical Research Associates (CRAs) can turn their attention to patient care and trial quality, knowing that AI is handling the data-heavy work.
One powerful AI application is in risk-based quality management (RBQM), particularly for fraud detection and the identification of "professional patients." Some individuals enroll in multiple trials for financial incentives or personal reasons, especially in high-demand studies like those for pain management or COVID-19 vaccines. AI can analyze enrollment patterns across sites, identify potential red flags, and prompt follow-ups for deeper investigation. This not only ensures trial integrity but also reduces reliance on time-consuming manual monitoring.
Beyond fraud, AI also excels at identifying subtle data inconsistencies that might otherwise be missed. Human patterns—such as habitual rounding or consistent data entry errors—can lead to skewed results. AI models can catch these errors quickly, cross-checking data across multiple trial sites to flag anomalies. By embedding AI in RBQM, clinical trials can improve data quality, leading to more reliable results and higher standards of study integrity.
In the coming years, as AI continues to accelerate trial design, patient recruitment, and data analysis, its true impact will be evident. The most transformative potential of AI isn’t just in speeding up individual processes but in enabling the scale of clinical trials without sacrificing quality or precision. By tapping into AI’s potential, contract research organizations (CROs) are better equipped to manage the rising complexity of global trials, bringing life-saving treatments to market faster and more efficiently than ever before. This shift represents a promising future for the life sciences, where technological advancements serve the highest purpose: improving patient outcomes and advancing global health.
Henry Lin is currently Senior Vice President, Data Science & AI at Emmes Group. Henry and his team build scalable data science and engineering software and services that are integrated into our platform to accelerate the generation of actionable insights across the clinical development lifecycle. Previously, Henry has led data science teams across different functions at Medidata Solutions, Janssen Pharmaceuticals, Johnson & Johnsons Medical Device Companies and Roche Pharmaceuticals. He earned his B.S. in Electrical Engineering & Computer Science from UC Berkeley and Ph.D. in Biological & Medical Informatics from UCSF. He can be reached at hlin@emmes.com.
Leave a comment
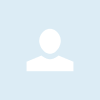
